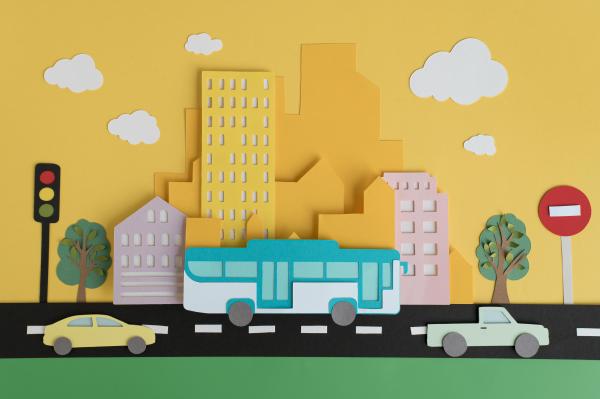
Societal impacts of Traffic
In today's urban landscapes, the staggering growth of traffic has become an alarming reality, with profound environmental and health implications. Studies indicate that excessive traffic contributes to a substantial increase in carbon emissions, accounting for approximately 25% of global greenhouse gas emissions. Moreover, the World Health Organization estimates that air pollution resulting from traffic congestion leads to more than 4 million premature deaths worldwide each year Additionally, noise pollution generated by traffic has been shown to exceed recommended levels in 70% of cities, affecting the quality of life of millions of urban dwellers. In the face of these daunting statistics, innovative solutions are urgently needed, such as the solutions proposed within the GreenMov project, including its traffic recommendation service – a promising avenue to mitigate the environmental impact of mobility. By harnessing advanced technologies and data-driven insights, these services aim to help city operators and citizens take decisions to optimize traffic flows, reduce congestion, potentially cut carbon emissions, and alleviate noise pollution levels.
Decoding Traffic Recommendations service
Current Smart cities are starting to harvest great amounts of data without having the opportunity to take advantage of all of them. In the case of traffic, cities are trying to find ways to help citizens in their effort to decarbonize their transport without truly knowing how to actually help them. Meanwhile, several mobility services exist (google maps, car sharing services, shared bikes, …) but they are usually not connected to each other and do not propose an all-in-one offering to the end-users. Furthermore, due to economic constraints that require companies to scale up in order to reduce their costs, such third party mobility services usually do not take advantage of local specificities or local sensors available. Greenmov aims to enable access to local data to any green mobility service provider. Furthermore, Greenmov provides its own green mobility service named the Traffic Recommendation service that aims to coordinate all other subservices developed within Greenmov. The strategy of such Traffic recommendation service consists in triggering forecasting services (air quality, noise, traffic density, …) and using a system expert approach to propose transport alternatives to the end-users.
A concrete example is the regulation of traffic depending on its environmental impact: when the traffic density forecast is high, the recommendation service will propose alternative ways to move, including the use of shared bikes if the weather forecast is good and bikes availability high, or the use of public transportation or shared cars in the other case. Similarly, another example relates to indications about the future availability of bikes at train stations to propose multimodal transportation solutions to end-users.
Deployment process of Fiware based traffic Recommendations service
Greenmov’s team worked on the process, the architecture and the functional solutions to allow traffic recommendations based on forecasts of multiple variables (traffic density, bikes availability, air quality, weather, noise, …). The deployment of a full and functional service requires to reproduce the different steps proposed in the image below. From left to right, replication of Greenmov’s services require a sensor infrastructure and connectors to send data in a standard and interoperable way leveraging NGSI-LD data models. The storage infrastructure of these data consists in any NGSI-LD compliant solution such as monitoring environmental parameters in a city or managing traffic on a specific area. For Greenmov use cases, the solution that was chosen was Orion-LD along with TimescaleDB for temporal data storage. An API manager can also be added in front of Orion-LD to change the API routes and control access to data. Then, Greenmov services based on Artificial Intelligence solutions such as timeseries forecasting allowed Greenmov’s engineering team to propose reliable traffic recommendations. Within Greenmov, engineers from Fiware Foundation, Atos-Eviden, IMEC, Libellium and the University of Nice Cote d’Azur have created new data models that go beyond the storage of measurements data and allow storage of forecasted values such as air quality or bikes availability forecasts. Finally, End-users can access these recommendations on demand, or be notified when these recommendations include a required change of behavior.
Feedbacks from end-users
The traffic recommendation engine described above has been implemented in Nice (France), Flanders and Murcia/Molina (Spain) and has been tested by friendly end-users. With a large majority of positive feedbacks, end-users would like to see such services integrated into their preferred mobility service interface such as “BlueBike” in Flanders, Google Maps or “Lignes d’Azur” in Nice. Then, end-users are paying attention to the quality and accuracy of the forecasts, and are asking for more forecasts, especially for a larger circle of prediction to not only limit the recommendations to the requested zone, but to increase the perimeter of the proposed service so they can decide by themselves the best option that meets their needs. This demonstrates the need for a user-driven integration of Greenmov solutions into Mobility service providers offerings.